Moscow Housing Analysis Model
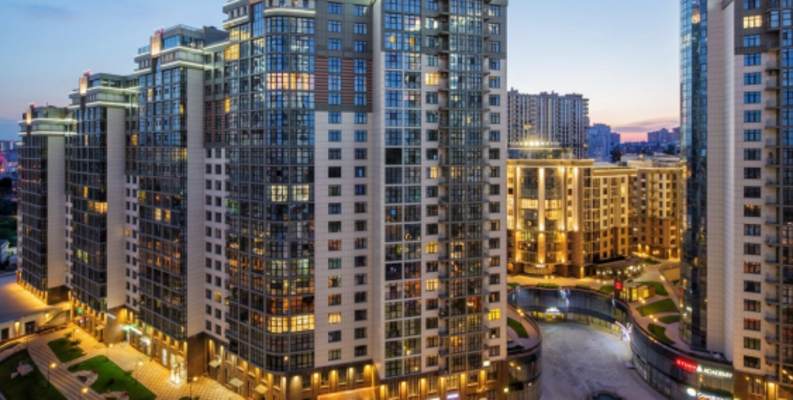
For further details, please refer to the GitHub repository linked here.
Moscow Housing Price Prediction Project
Overview
The Moscow Housing Price Prediction Project aims to develop a robust machine learning model for predicting housing prices in Moscow and the Moscow Oblast region. Leveraging a comprehensive dataset containing essential attributes such as location, size, amenities, and renovation level, the project endeavors to provide valuable insights into the dynamic real estate market in this area.
Dataset
The dataset utilized for this project, collected in November 2023, encompasses crucial information essential for predicting housing costs. It includes the following columns:
- Price: Price of the apartment
- Apartment type: Type of apartment (e.g., studio, one-bedroom, etc.)
- Metro station: Nearest metro station
- Minutes to metro: Time in minutes to the nearest metro station
- Region: Region where the apartment is located (Moscow or Moscow Oblast)
- Number of rooms: Total number of rooms in the apartment
- Area: Total area of the apartment in square meters
- Living area: Living area of the apartment in square meters
- Kitchen area: Area of the kitchen in square meters
- Floor: Floor on which the apartment is located
- Number of floors: Total number of floors in the building
- Renovation: Level of renovation of the apartment
Project Structure
The project adheres to a structured organization, facilitating ease of navigation and understanding. Key components include:
- data/: Directory housing dataset files and new datasets with interaction features.
- model/: Directory for saving and loading trained models.
- dashboard.py: Python script for creating a dashboard using Vizro to visualize scatter and histogram plots.
- requirements.txt: File containing the required dependencies for running the project.
- README.md: Comprehensive documentation providing an overview of the project, its structure, and usage instructions.
Analysis Process
The project undertook a systematic analysis process, comprising the following steps:
- Data Exploration: Comprehensive exploration of the dataset’s structure, examination of summary statistics, and exploration of housing price distribution and other key attributes.
- Data Preprocessing: Handling of categorical variables through one-hot encoding and splitting the dataset into training and testing sets.
- Initial Model Building: Training of a Random Forest regression model on the original features and evaluation of its performance using mean squared error (MSE) and mean absolute error (MAE).
- Feature Engineering: Creation of interaction features between relevant variables to capture potential nonlinear relationships or interactions.
- Hyperparameter Tuning: Conducting grid search using cross-validation to identify the best hyperparameters for the Random Forest model.
- Model Optimization: Saving the best-performing model for future use and validation.
- Model Evaluation: Assessment of model performance using R-squared and visualizations for comparison of actual vs. predicted values.
Latest Update
The project has undergone recent enhancements, including:
- Addition of interaction features between key variables to capture nuanced relationships.
- Refitting of the Random Forest model on updated training data and evaluation of its performance.
- Hyperparameter tuning using GridSearchCV to further optimize the model.
- Saving of the optimized model for future deployment and validation.
How to Use
To utilize the project:
- Install the required dependencies using
pip install -r requirements.txt
. - Run
dashboard.py
to visualize scatter and histogram plots using Vizro and open your browser onlocalhost:8050
.
Moscow Housing Dashboard
- Access trained models from the
model/
directory for predictions. - Explore datasets in the
data/
directory for further analysis.
Acknowledgments
- The project draws inspiration from the Moscow Housing Price Dataset available on Kaggle.
- Special thanks to Vizro for providing tools to create interactive dashboards for data visualization.
Author
- Mohammed Mebarek Mecheter
- Contact: mohammedmecheter@gmail.com
- LinkedIn: Mohammed Mebarek Mecheter
- GitHub: Mohammed Mebarek Mecheter
Contributing
Contributions to the project are encouraged. For issues or suggestions, please open an issue or submit a pull request. Your feedback is valued and appreciated.